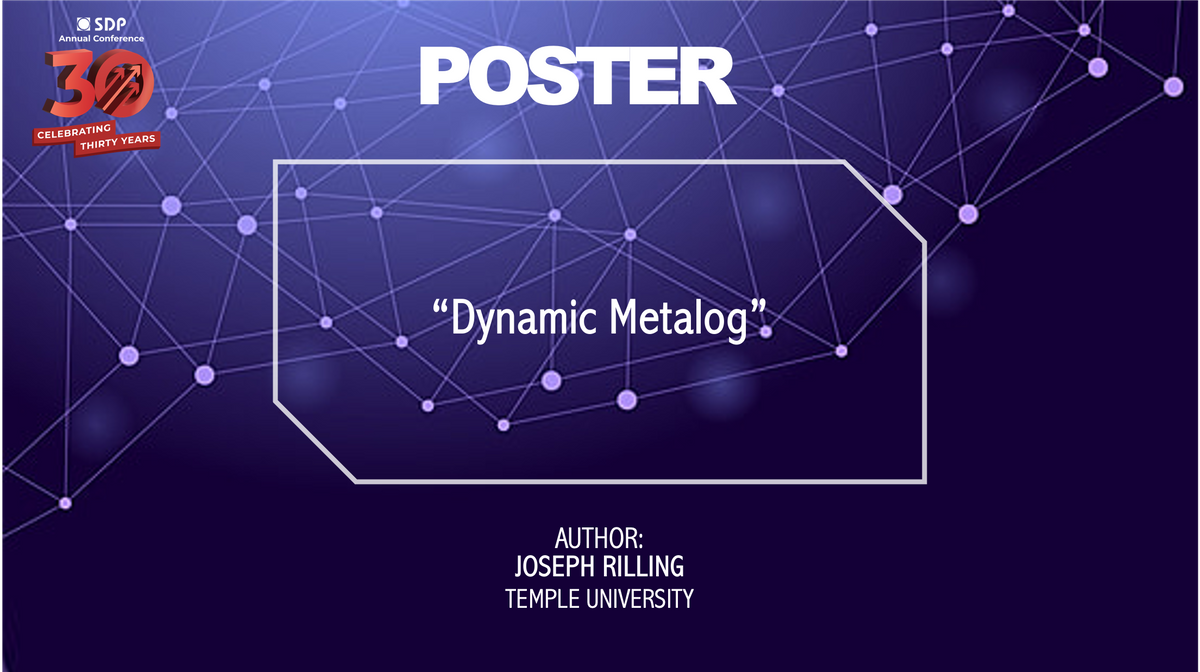
DYNAMIC METALOG
Author: Joseph Rilling (Temple University)
Abstract:
The recently introduced metalog distributions (Keelin 2016) present an alternative to traditional probability distributions. Metalog distributions are extremely flexible and can be easily fit via ordinary least squares estimation. Once fit, a metalog distribution immediately yields a CDF, PDF, and quantile function, making decision analysis and monte carlo methods trivial.
The metalog procedure needs to estimate the percentile of each observation. All current methods calculate the percentiles by implicitly assuming observations are independently and identically distributed from the same static population. This assumption is not always met, as populations naturally evolve with time. Keelin and Howard 2021 presents a Bayesian updating procedure, which works well when using information gathered from one population to help fit a second population. However, this procedure does not address the deeper and more nuanced problem of continuously evolving populations.
This work presents the dynamic metalog, a novel way to extend metalogs to settings with continuously evolving populations. This new method is a latent variable dependent dynamic linear model and presents a new outlook on population time series. The dynamic metalog treats the percentiles as unknown latent variables and can sample from the joint distribution of the latent percentiles and the model parameters. Starting with fishing data from the rmetalog package (Faber and Jung 2021), we create several examples of evolving populations. In these simulations, the dynamic metalog demonstrates the ability to adapt to changes in location, scale, and both at the same time.